Paper in IEEE WACV (2017): "Complex Event Recognition from Images with Few Training Examples"
Paper
- U. Ahsan, C. Sun, J. Hays, and Irfan Essa (2017), “Complex Event Recognition from Images with Few Training Examples,” in IEEE Winter Conference on Applications of Computer Vision (WACV), 2017. [PDF] [arXiv] [BIBTEX]
@InProceedings{ 2017-Ahsan-CERFIWTE, arxiv = {https://arxiv.org/abs/1701.04769}, author = {Unaiza Ahsan and Chen Sun and James Hays and Irfan Essa}, booktitle = {{IEEE Winter Conference on Applications of Computer Vision (WACV)}}, month = {March}, pdf = {http://www.cc.gatech.edu/~irfan/p/2017-Ahsan-CERFIWTE.pdf}, title = {Complex Event Recognition from Images with Few Training Examples}, year = {2017} }
Abstract
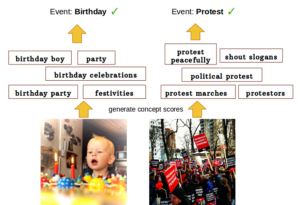
We propose to leverage concept-level representations for complex event recognition in photographs given limited training examples. We introduce a novel framework to discover event concept attributes from the web and use that to extract semantic features from images and classify them into social event categories with few training examples. Discovered concepts include a variety of objects, scenes, actions and event subtypes, leading to a discriminative and compact representation for event images. Web images are obtained for each discovered event concept and we use (pre-trained) CNN features to train concept classifiers. Extensive experiments on challenging event datasets demonstrate that our proposed method outperforms several baselines using deep CNN features directly in classifying images into events with limited training examples. We also demonstrate that our method achieves the best overall accuracy on a data set with unseen event categories using a single training example.
- Presented at IEEE and PAMITC’s Winter Conference on Applications of Computer Vision, March 27-30, 2017, in Santa Rosa, CA, USA.