Paper in IPCAI 2017 on "Video and Accelerometer-Based Motion Analysis for Automated Surgical Skills Assessment"
Paper
- A. Zia, Y. Sharma, V. Bettadapura, E.Sarin, and I. Essa (2017), “Video and Accelerometer-Based Motion Analysis for Automated Surgical Skills Assessment,” in Information Processing in Computer-Assisted Interventions (IPCAI), 2017. [PDF] [BIBTEX]
@InProceedings{ 2017-Zia-VAMAASSA, author = {A. Zia and Y. Sharma and V. Bettadapura and E.Sarin and I. Essa}, booktitle = {{Information Processing in Computer-Assisted Interventions (IPCAI)}}, month = {June}, pdf = {http://www.cc.gatech.edu/~irfan/p/2017-Zia-VAMAASSA.pdf}, title = {Video and Accelerometer-Based Motion Analysis for Automated Surgical Skills Assessment}, year = {2017} }
Abstract
Purpose: Basic surgical skills of suturing and knot tying are an essential part of medical training. Having an automated system for surgical skills assessment could help save experts time and improve training efficiency. There have been some recent attempts at automated surgical skills assessment using either video analysis or acceleration data. In this paper, we present a novel approach for automated
assessment of OSATS based surgical skills and provide an analysis of different features on multi-modal data (video and accelerometer data).
Methods: We conduct the largest study, to the best of our knowledge, for basic surgical skills assessment on a dataset that contained video and accelerometer data for suturing and knot-tying tasks. We introduce “entropy-based” features – Approximate Entropy (ApEn) and Cross-Approximate Entropy (XApEn), which quantify the amount of predictability and regularity of fluctuations in time-series data. The
proposed features are compared to existing methods of Sequential Motion Texture (SMT), Discrete Cosine Transform (DCT) and Discrete Fourier Transform (DFT), for surgical skills assessment.
Results: We report the average performance of different features across all applicable OSATS criteria for suturing and knot tying tasks. Our analysis shows that the proposed entropy-based features out-perform previous state-of-the-art methods using video data. For accelerometer data, our method performs better for suturing only. We also show that fusion of video and acceleration features can improve overall performance with the proposed entropy features achieving the highest accuracy.
Conclusions: Automated surgical skills assessment can be achieved with high accuracy using the proposed entropy features. Such a system can significantly improve the efficiency of surgical training in medical schools and teaching hospitals.
- Presented at The 8th International Conference on Information Processing in Computer-Assisted Interventions, in Barcelona, SPAIN, June 20-21, 2017.
- Aneeq Zia awarded the “Young Investigator Travel Award” given to young investigators (including Ph.D. and MSc students and junior researchers) with accepted papers at IPCAI conference to attend IPCAI/CARS 2017.
- This paper was also 1 of the 12 papers voted by the audience for a 25 minute long oral presentation and discussion session on the last day of conference (based on 5 minute short presentations given by all authors on the first day).
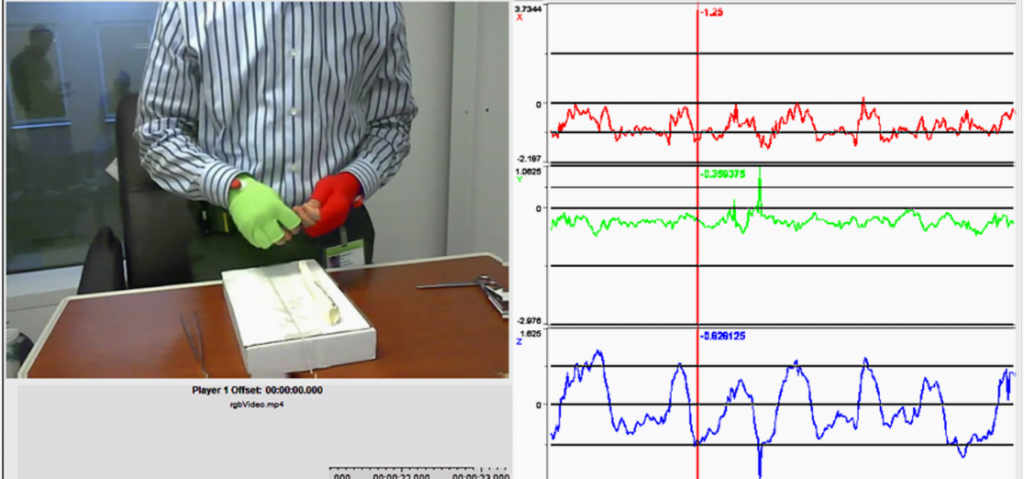